I think I finally start to understand what is the time. If I understand correctly, time does not even exist. It is just a measure of change. Nobody cares about time, other than some living creatures. And that is because we want to be alive, to remain in our integrality or to maintain our identity. What if we don't exist? Time is there? I think not. Change is there, but not time. It looks like time is only a measurement. This is why it "flows" only forward.
Broken thoughts
data, ml, stat, life, physics, maths, philosophy
September 24, 2021
September 19, 2021
Java 17 GA: Simple benchmark with Vector API (Second Preview)
A few years ago I was hoping that Java will have a chance to become again an important contented into machine learning field. I was hoping for interactivity, vectorization, and seamless integration with the external world (c/c++/fortran). With the last release of Java 17 the last two dreams are closer to reality than ever.
JEP 414: Vector API (Second Incubator) is something I awaited a lot and I spent a few hours playing with it. Personally, I am really happy with the results, and I have a lot of motivation to migrate much of the linear algebra staff on that. It looks really cool.
To make a story short, I implemented a small set of microbenchmarks for two simple operations. The first operation is fillNaN and for the second test, we simply add elements of a vector.
fillNaN
This is a common problem when working with large chunks of floating numbers: some of them are not numbers for various reasons: missing data, impossible operations, and so on. A panda version of it could be fillna. The whole idea is that for a given vector you want to replace all Double.NaN values with a given value to make arithmetic possible.
The following is a listing of the fillNa benchmark.
As you can see, nothing fancy here. The `testFillNaNArrays` method iterates over the array and if the given value is Double.NaN. Pretty straightforward. How about the results? It should be faster.
Benchmark Mode Cnt Score Error Units
VectorFillNaNBenchmark.testFillNaNArrays thrpt 10 3.405 ± 0.149 ops/ms
VectorFillNaNBenchmark.testFillNaNVectorized thrpt 10 41.930 ± 4.437 ops/ms
VectorFillNaNBenchmark.testFillNaNArrays avgt 10 0.289 ± 0.002 ms/op
VectorFillNaNBenchmark.testFillNaNVectorized avgt 10 0.023 ± 0.001 ms/op
But over 10 times faster? It is a really pleasant surprise, but not quite a surprise. This is in strict connection with auto-vectorization in Java. When it works, and for simple loops it works, it gives intrinsic optimizations and sometimes even SIMD based. But calling such a thing as Double.isNaN is not a simple thing, at least for auto-vectorization. In the new Vector API this operation is vectorized and we go fast, even if we use masks, which are not the lightest things in this new API. So we get a boost of 13x in speed which looks amazing.
sum and sumNaN
For the second microbenchmark, we have the same operation in two flavors. The first sum is implemented over all elements, with no constraints. The second sum operation, we call it sumNaN skips the potential non-numeric values and computes the sum of the rest of the numbers. We do that to check two things. We want to know how vectorization behaves compared to auto-vectorization (this is the normal sum, which is implemented as a simple loop that benefits from all optimizations possible). And we also want to see another operation with masks, compared with an auto-vectorized code. Let's see the benchmark:
Conclusions
June 7, 2016
z test in rapaio library
Hypothesis testing
Z tests
Example 1: One sample z-test
355.02 355.47 353.01 355.93 356.66 355.98 353.74 354.96 353.81 355.79
(
// build the sample
Var cans = Numeric.copy(355.02, 355.47, 353.01, 355.93, 356.66, 355.98, 353.74, 354.96, 353.81, 355.79);
// run the test and print results
HTTools.zTestOneSample(cans, 355, 0.05).printSummary();
> HTTools.zTestOneSample
One Sample z-test
mean: 355
sd: 0.05
significance level: 0.05
alternative hypothesis: two tails P > |z|
sample size: 10
sample mean: 355.037
z score: 2.3400855
p-value: 0.019279327322640594
conf int: [355.0060102,355.0679898]
- the z-score is
2.34 , which means that the computed sample mean is greater with more than 2 standard deviations - for critical level being
0.05 and p-value0.019 , we reject the null hypothesis that the mean volume delivered by the machine is equal with355
What if we ask if the machine produces more than standard specification?
HTTools.zTestOneSample(cans,
355, \\ mean
0.05, \\ sd
0.05, \\ significance level
HTTools.Alternative.GREATER_THAN \\ alternative
).printSummary();
> HTTools.zTestOneSample
One Sample z-test
mean: 355
sd: 0.05
significance level: 0.05
alternative hypothesis: one tail P > z
sample size: 10
sample mean: 355.037
z score: 2.3400855
p-value: 0.009639663661320297
conf int: [355.0060102,355.0679898]
Example 2: One sample z-test
ZTestOneSample ztest = HTTools.zTestOneSample(
6.7, // sample mean
29, // sample size
5, // tested mean
7.1, // population standard deviation
0.05, // significance level
HTTools.Alternative.GREATER_THAN // alternative
);
ztest.printSummary();
> HTTools.zTestOneSample
One Sample z-test
mean: 5
sd: 7.1
significance level: 0.05
alternative hypothesis: one tail P > z
sample size: 29
sample mean: 6.7
z score: 1.2894057
p-value: 0.0986285477062051
conf int: [4.1159112,9.2840888]
Example 3: Two samples z test
HTTools.zTestTwoSamples(
28, 75, // male sample mean and size
33, 50, // female sample mean and size
0, // difference of means
14.1, 9.5, // standard deviations
).printSummary();
> HTTools.zTestTwoSamples
Two Samples z-test
x sample mean: 28
x sample size: 75
y sample mean: 33
y sample size: 50
mean: 0
x sd: 14.1
y sd: 9.5
significance level: 0.05
alternative hypothesis: two tails P > |z|
sample mean: -5
z score: -2.3686842
p-value: 0.017851489594360337
conf int: [-9.1372421,-0.8627579]
January 20, 2016
Tutorial on Kaggle's Titanic Competition using rapaio
You can read the tutorial here.
The manual for rapaio library can be found here.
The code repository can be found here.
Let me know if you like it.
January 11, 2016
Rapaio Manual - Graphics: Histograms
Histogram
Example 1
sepal-length
variable from iris
data set. WS.draw(hist(iris.var("sepal-length")));
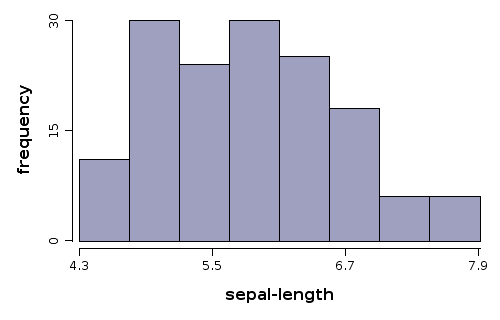
Example 2
sepal-length
and petal-length
variables from iris
data set. We want to get bins in range (0-10) of width 0.25, colored with red, and blue, with a big transparency for visibilityWS.draw(plot(alpha(0.3f))
.hist(iris.var("sepal-length"), 0, 10, bins(40), color(1))
.hist(iris.var("petal-length"), 0, 10, bins(40), color(2))
.legend(7, 20, labels("sepal-length", "petal-length"), color(1, 2))
.xLab("variable"));
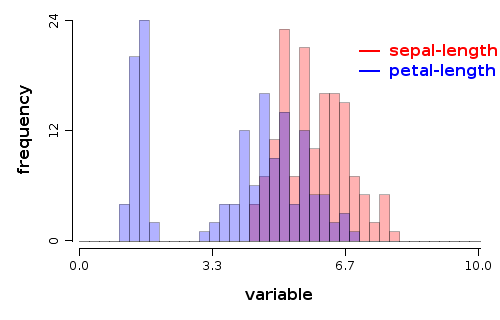
plot(alpha(0.3f))
- builds an empty plot; this is used only to pass default values for alpha for all plot components, otherwise the plot construct would not be neededhist
- adds a histogram to the current plotiris.var("sepal-length")
- variable used to build histogram0, 10
- specifies the range used to compute binsbins(40)
- specifies the number of bins for histogramcolor(1)
- specifies the color to draw the histogram, which is the color indexed with 1 in color palette (in this case is red)legend(7, 20, ...)
- draws a legend at the specified coordinates, values are in the units specified by datalabels(..)
- specifies labels for legendcolor(1, 2)
- specifies color for legendxLab
= specifies label text for horizontal axis